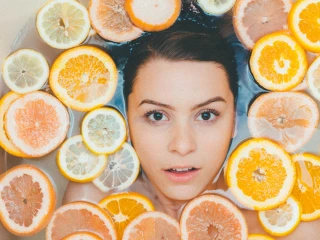
Unlocking the potential of data science in retail at Distriplus
- operations
- sales, marketing and service
- retail
What if we could orchestrate online and offline customer behavior with data science? For many retailers, that dream will probably remain a dream for the foreseeable future. Beauty and care retailer Distriplus (Di, Planet Parfum) decided to make it a reality – now. Together with delaware, the company built a proof of concept that would allow it to analyze buying patterns and detect cross-selling opportunities, not online but in its brick and mortar stores.